Effective Data Validation with Sigma Data Systems
There are plenty of methods and ways to validate data, such as employing validation rules and constraints, establishing routines and workflows, and checking and reviewing data.
For this article, we are guiding for best practices for the data validation system to adapt when inspecting for data that we used successfully.
Till the date, we are not surprised by errors to be found in data every time with the absolute quantity at times from resource to multinational businesses and caused for a complete variety of reasons.
When blending various data sources to a single data repository such as a data warehouse or data lack, a short of consistency is already a real problem created by errors.
What is data validation?
When data is collected, it may not be favorable, if not checked properly. Now, to make sure of data quality and its usability, the validation process takes place. For instance, if your collected data is unsorted or say dreadful, business units will be uncertain about making decisions with it and its end with unused data.
Now the question arises to trust the gathered data for the useful insights or not?
- The mistake codes spread into the serving information, and everything looks typical. And for the same, data validation using machine learning helps to deal with errors.
- The serving information, in the long run, becomes preparing information, and the AI and ML model helps to figure out data validation and how to predict the component esteem.
The age of the information decoupled in the model that is outlined as a unique arrangement. An absence of visibility by the ML pipeline into this information age rationale except symptoms makes distinguishing such cut explicit issues fundamentally harder.
Mistakes brought about by bugs in code are reasonable, and will, in general, be not the same as that sort of blunders usually considered in the information cleaning writing.
Consider 900+ pages of data on one person
At the point when a developer, as of late, got 900 pages of data about a year as a tolerably substantial client of an eCommerce, tracking, or dating site, a great many people, just remained with the idea of how much information one site had on one individual.
We realize that organizations basically can’t bear to get their information wrong, regardless of whether it is for legitimate consistency, showing on a site, gathered from Internet of Things sensors or utilized for promoting and deals battles and inward business knowledge and details.
However, as of one year from now, inside the European Union, such information must be given lawfully to any individual who requires it, and this is a pattern that is more than liable to expand around the world.
The question that emerges here is what number of blunders were there inside those 900+ pages of information?
Not excessively, we feel that connecting locales are bound to deliver information mistakes more than some other organizations. Without a doubt, they might be one of those minorities of organizations which as of now have the procedures set up to successfully approve their information.
These ensures that they are a sans mistake, not simply for this one client however the huge number if not a great many clients they have on their books.
However, neither has it showed up did the columnist experience those 900 pages with a fine-tooth comb searching for blunders?
For instance, if someone managed to do things as such to discover maverick information blunders that damaged a portion of these critical new information and information security laws, for example, GDPR, it could have given that organization significant issues.
Integrating data validation in Machine Learning
For machine learning process flow, Data validation is an important part. Google uses data validation for its ML processing.
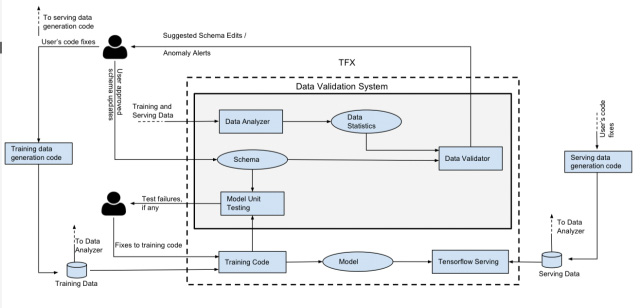
Process flow conventionally works constantly with the presence of another bunch of information setting off another run.
The pipeline ingests the readiness data, favors it, sends it to an arrangement estimation to make a model, and a short time later drives the readied model to a serving establishment for acceptance.
Here the data endorsement sorts out has three central parts:
• The data support checks properties of the data against an example,
• The data analyzer forms bits of knowledge over the new data bunch,
• The model unit analyzer looks for errors in the ready code using fabricated data.
Detecting Slant
A couple of strangeness potentially shows up when taking a validation at data across different gatherings; for example, incline among getting ready and serving data.
• Feature incline happens when a particular component acknowledges different characteristics in getting prepared instead of investing energy in prison. For example, a planner may have included or ousted a segment.
Or then again harder to recognize, data may be gotten by calling a period fragile API, for instance, the recuperating the number of gobbles up until this point, and the sneaked past time could be particular in getting ready and serving.
• Distribution slant happens when the course of feature regards over a bunch of getting available data is novel comparable to that seen at investing energy in prison.
For example, examining the present data is used for setting up the next day’s model, and there is a bug in the testing code.
• Scoring/serving incline happens when the way wherein results are acquainted with the customer can analyze into the planning data.
For example, scoring one hundred accounts, yet simply demonstrating the first ten. The other ninety won’t get any snaps.
Benefits of Data Validation with the Sigma Data Systems
For any of your requirements, you can go through Sigma Data systems to get a world-class solution with your historical data. We take care of clients’ data on priority notes while they are in our care. We guarantee that your business data is not accessible by any third party, a responsibility we take gravely.
⦁ Validating your data helps you to enhance competence while generating leads and developing lead funnels.
⦁ Data validation especially helps to increase sales channel efficiency. And saves time by parting incorrect data from the data slot.
⦁ It helps your server to restrict from processing malicious code (SQL injection attack) as a security point of view and can save the whole database from being hacked.
⦁ It is the process of data cleaning and removes incorrect data to get better insights and increase data reliability.
Quality of data matters to us, and we will help your business to stand out for various resources. For the same, data cleaning helps along with validation to bifurcate data from waste and helps to make improved decisions for the business process.